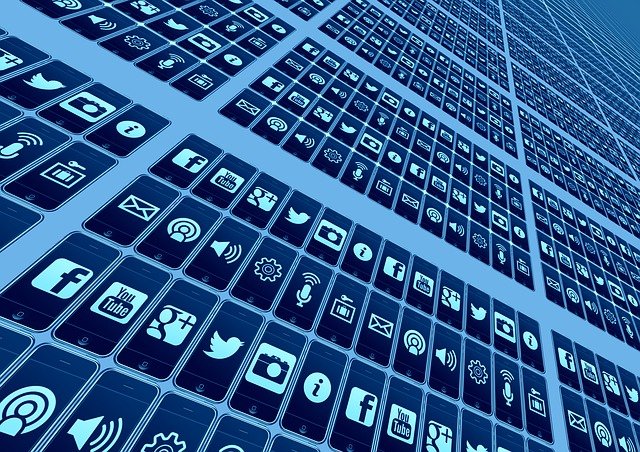
Following Red Dress Day on May 5, a day aimed to raise awareness for Missing and Murdered Indigenous Women and Girls (MMIWG), Indigenous activists and supporters of the campaign found posts about MMIWG had disappeared from their Instagram accounts. In response, Instagram released a tweet saying that this was “a widespread global technical issue not related to any particular topic,” followed by an apology explaining that the platform “experienced a technical bug, which impacted millions of people’s stories, highlights and archives around the world.”
Creators, however, said that not all stories were affected.
And this is not the first time social media platforms have been under scrutiny because of their erroneous censoring of grassroots activists and racial minorities.
Many Black Lives Matter (BLM) activists were similarly frustrated when Facebook flagged their accounts, but didn’t do enough to stop racism and hate speech against Black people on their platform.
So were these really about technical glitches? Or did they result from the platforms’ discriminatory and biased policies and practices? The answer lies somewhere in between.
Towards automated content moderation
Every time an activist’s post is wrongly removed, there are at least three possible scenarios.
First, sometimes the platform deliberately takes down activists’ posts and accounts, usually at request of and/or in co-ordination with the government. This happened when Facebook and Instagram removed posts and accounts of Iranians who expressed support for the Iranian general Qassem Soleiman.
In some countries and disputed territories, such as Kashmir, Crimea, Western Sahara and Palestinian territories, platforms censored activists and journalists to allegedly maintain their market access or to protect themselves from legal liabilities.
Second, a post can be removed through a user-reporting mechanism. To handle unlawful or prohibited communication, social media platforms have indeed primarily relied on users reporting.
Applying community standards developed by the platform, content moderators would then review reported content and determine whether a violation had occurred. If it had, the content would be removed, and, in the case of serious or repeat infringements, the user may be temporarily suspended or permanently banned.
This mechanism is problematic. Due to the sheer volume of reports received on a daily basis, there are simply not enough moderators to review each report adequately. Also, complexities and subtleties of language pose real challenges. Meanwhile, marginalized groups reclaiming abusive terms for public awareness, such as BLM and MMIWG, can be misinterpreted as being abusive.
Further, in flagging content, users tend to rely on partisanship and ideology. User reporting approach is driven by popular opinion of a platform’s users while potentially repressing the right to unpopular speech.
Such approach also emboldens freedom to hate, where users exercise their right to voice their opinions while actively silencing others. A notable example is the removal by Facebook of “Freedom for Palestine,” a multi-artist collaboration posted by Coldplay, after a number of users reported the song as “abusive.”
Third, platforms are increasingly using artificial intelligence (AI) to help identify and remove prohibited content. The idea is that complex algorithms that use natural language processing can flag racist or violent content faster and better than humans possibly can. During the COVID-19 pandemic, social media companies are relying more on AI to cover for tens of thousands of human moderators who were sent home. Now, more than ever, algorithms decide what users can and cannot post online.
Algorithmic biases
There’s an inherent belief that AI systems are less biased and can scale better than human beings. In practice, however, they’re easily disposed to error and can impose bias on a colossal systemic scale.
In two 2019 computational linguistic studies, researchers discovered that AI intended to identify hate speech may actually end up amplifying racial bias.
In one study, researchers found that tweets written in African American English commonly spoken by Black Americans are up to twice more likely to be flagged as offensive compared to others. Using a dataset of 155,800 tweets, another study found a similar widespread racial bias against Black speeches.
What’s considered offensive is bound to social context; terms that are slurs when used in some settings may not be in others. Algorithmic systems lack an ability to capture nuances and contextual particularities, which may not be understood by human moderators who test data used to train these algorithms either. This means natural language processing which is often perceived as an objective tool to identify offensive content can amplify the same biases that human beings have.
Algorithmic bias may jeopardize some people who are already at risk by wrongly categorizing them as offensive, criminals or even terrorists. In mid 2020, Facebook deleted at least 35 accounts of Syrian journalists and activists on the pretext of terrorism while in reality, they were campaigning against violence and terrorism.
MMIWG, BLM and the Syrian cases exemplify the dynamic of “algorithms of opression” where algorithms reinforce older oppressive social relations and re-install new modes of racism and discrimination.
While AI is celebrated as autonomous technology that can develop away from human intervention, it is inherently biased. The inequalities that underpin bias already exist in society and influence who gets the opportunity to build algorithms and their databases, and for what purpose. As such, algorithms do not intrinsically provide ways for marginalized people to escape discrimination, but they also reproduce new forms of inequality along social, racial and political lines.
Despite the apparent problems, algorithms are here to stay. There is no silver bullet, but one can take steps to minimize bias. First is to recognize that there’s a problem. Then, making a strong commitment to root out algorithmic biases.
Bias can infiltrate the process anywhere in designing algorithms.
The inclusion of more people from diverse backgrounds within this process — Indigenous, racial minorities, women and other historically marginalized groups — is one of important steps to help mitigate the bias. In the meantime, it is important to push platforms to allow for as much transparency and public oversight as possible.
By Merlyna Lim
Canada Research Chair in Digital Media & Global Network Society and Founding Director of ALiGN Media Lab, Carleton University
By Ghadah Alrasheed
Post-doctoral Fellow, Interim co-Director of ALiGN Media Lab, Carleton University